On November 20, a groundbreaking study by Professor Chang Lingqian’s team from the School of Biological Science and Medical Engineering at Beihang University and Professor Yu Xinge’s team from City University of Hong Kong, along with collaborating teams from the University of Hong Kong and West China Hospital of Sichuan University, was published in Nature Communications. Dr. Li Hu (Beihang University/City University of Hong Kong) and Dr. Wang Yuqiong (Beihang University) are co-first authors. Prof. Chang Lingqian, Prof. Huang Jiandong (University of Hong Kong), and Prof. Hu Wenchuang (West China Hospital of Sichuan University) are co-corresponding authors of this paper.
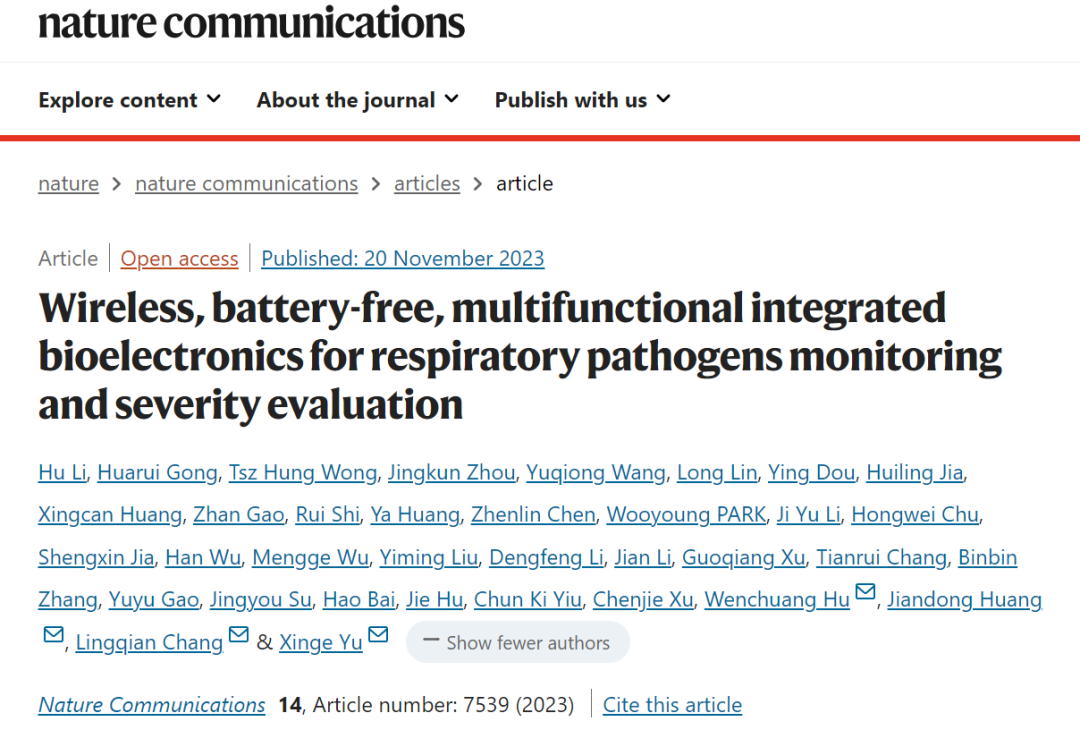
Their findings, titled “Wireless, battery-free, multifunctional integrated bioelectronics for respiratory pathogens monitoring and severity evaluation”, reported a wireless, battery-free, multifunctional pathogenic infection diagnosis system (PIDS) that can accurately capture and detect respiratory pathogens, and provide real-time evaluation of infection severity with the assistance of machine learning.
The unresolved technical barriers exist in the rapid collection and onsite identification of the exhaled SARS-CoV-2 by the platform itself, multi-parameter monitoring and in-depth analysis, miniaturized system integration and non-invasive severity assessment after virus infection.
The researchers developed a gas-liquid interface aerosol microchannel structure as a solution to those challenges to achieve continuous and rapid capture of the virus at the gas-liquid interface. By integrating flexible bioelectronics encompassing immune biosensor (IBS) module, respiration rate sensor, temperature sensor (TBS) module, and near-field communication (NFC) wireless communication chips, along with the use of machine learning technology, the researchers achieved rapid and accurate diagnosis of SARS-CoV-2 infection and its associated symptom severity through respiratory activities. Moreover, by making appropriate structural adjustments, the researchers demonstrated the application of this technology in miniaturized wearable electronic diagnostic devices, personalized electronic diagnostic accessories, and skin-mounted electronic devices (see Fig.1).
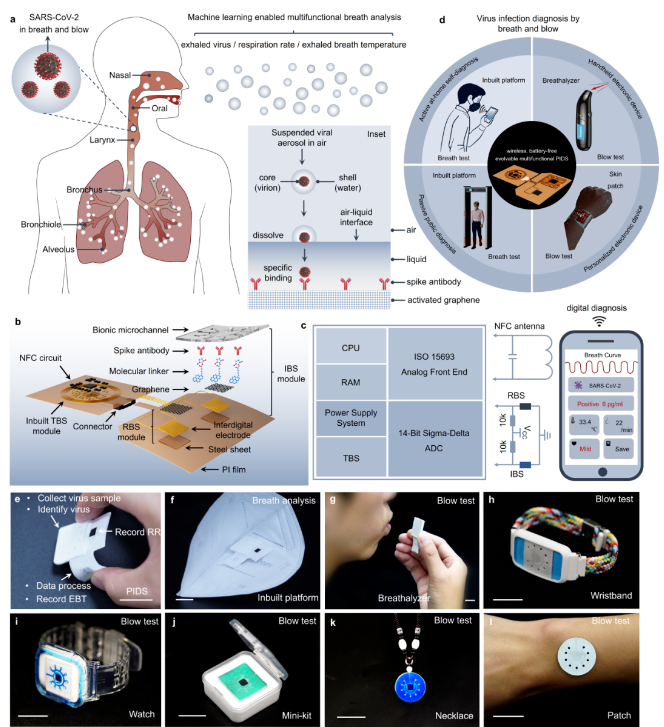
Fig. 1 Wireless, battery-free, multifunctional PIDS for diagnosing virus infection by breath and blow
A total of 42 volunteers were recruited in the experiment, and the results indicated that the technology could achieve diagnosis of positive and negative cases within 1 min. The accuracy for blow test reached 100% at 4 min, and the accuracy for breath test reached 100% at 25 min. The rapid diagnostic results of SARS-CoV-2 infection were transmitted and analyzed via smartphone NFC wireless breath tests (see Fig. 2).
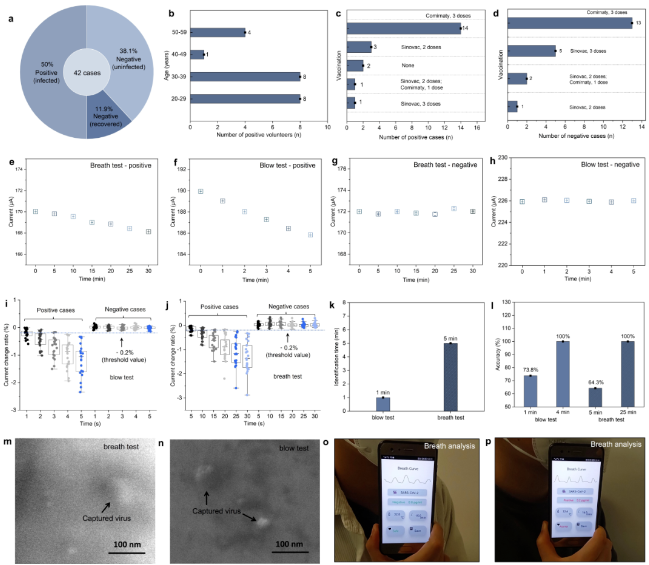
Fig. 2 Rapid and accurate identification of positive and negative cases by breath and blow tests
Furthermore, the researchers integrated machine learning into the system to evaluate viral infection and symptom severity, and the experimental results demonstrated a high degree of consistency between the machine learning evaluations and the actual conditions of the infected individuals (see Fig. 3).
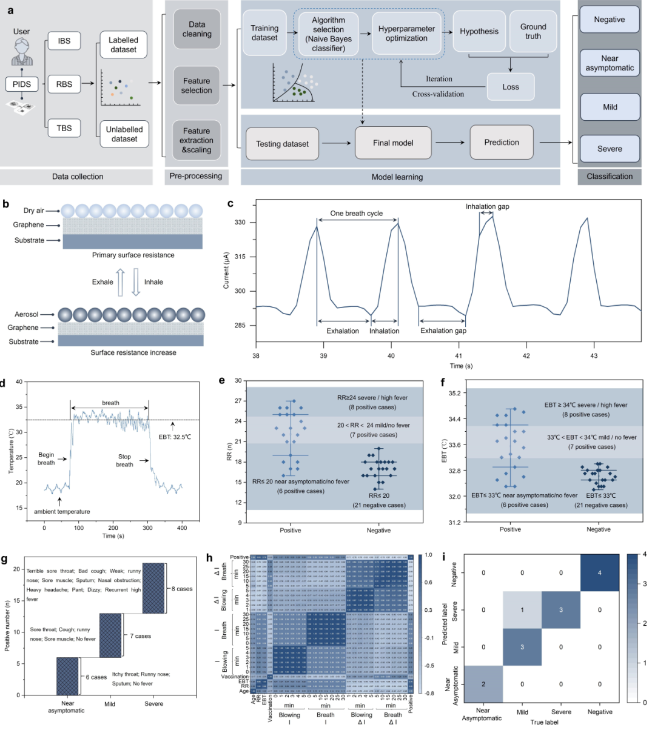
Fig. 3 Machine learning-enabled multiparameter breath analysis for diagnosing SARS-CoV-2 infection and evaluating symptom severity
Eliminating the need for manual sample collection, complex sample pretreatment, expensive large-scale equipment, frequent battery replacements, and tedious laboratory testing procedures, this general-purpose technology is equally applicable to the detection of the current widespread influenza virus.
Written by: Ni Yikun
Reviewed by: Li Deyu
Edited by: Jia Aiping
Translated by: Han Xu