Recently, the research team led by Prof. Yang Lijun and Prof. Deng Yue from Beihang University, in collaboration with Professor Sun Hao’s team from Renmin University of China, published an article titled "Learning spatiotemporal dynamics with a pretrained generative model" in Nature Machine Intelligence, a sub-journal of Nature.
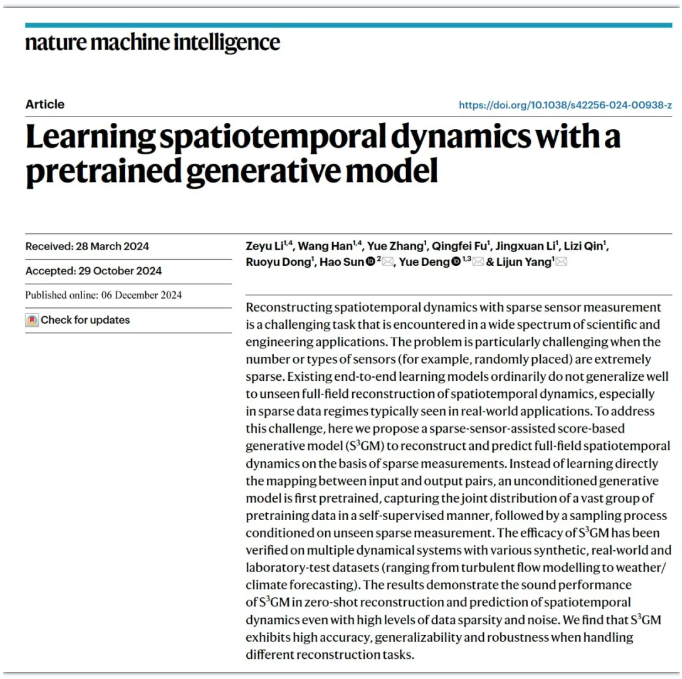
Li Zeyu, a doctoral student supervised by Prof. Yang Lijun, and Prof. Han Wang from Beihang University are the first authors. Prof. Yang Lijun, Prof. Deng Yue, and Prof. Sun Hao are the corresponding authors. The School of Astronautics at Beihang University is the primary affiliation for the study.
Reconstructing spatiotemporal dynamics with sparse sensor measurement is a challenging task that is encountered in a wide spectrum of scientific and engineering applications. The problem is particularly challenging when the number or types of sensors (for example, randomly placed) are extremely sparse. Existing end-to-end learning models ordinarily do not generalize well to unseen full-field reconstruction of spatiotemporal dynamics, especially in sparse data regimes typically seen in real-world applications.
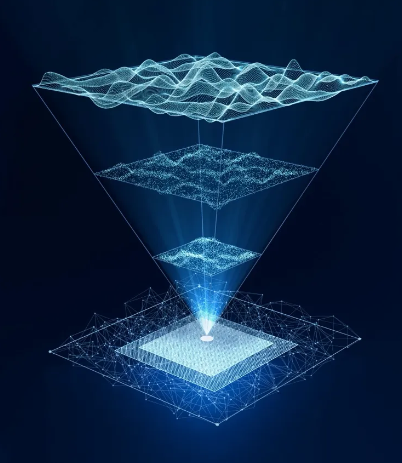
To address this challenge, the researchers propose a sparse-sensor-assisted score-based generative model (S3GM) to reconstruct and predict full-field spatiotemporal dynamics based on sparse measurements. Instead of learning directly the mapping between input and output pairs, an unconditioned generative model is first pretrained, capturing the joint distribution of a vast group of pretraining data in a self-supervised manner, followed by a sampling process conditioned on unseen sparse measurement. The efficacy of S3GM has been verified on multiple dynamical systems with various synthetic, real-world and laboratory-test datasets (ranging from turbulent flow modelling to weather/climate forecasting). The results demonstrate the sound performance of S3GM in zero-shot reconstruction and prediction of spatiotemporal dynamics even with high levels of data sparsity and noise. S3GM exhibits high accuracy, generalizability and robustness when handling different reconstruction tasks.
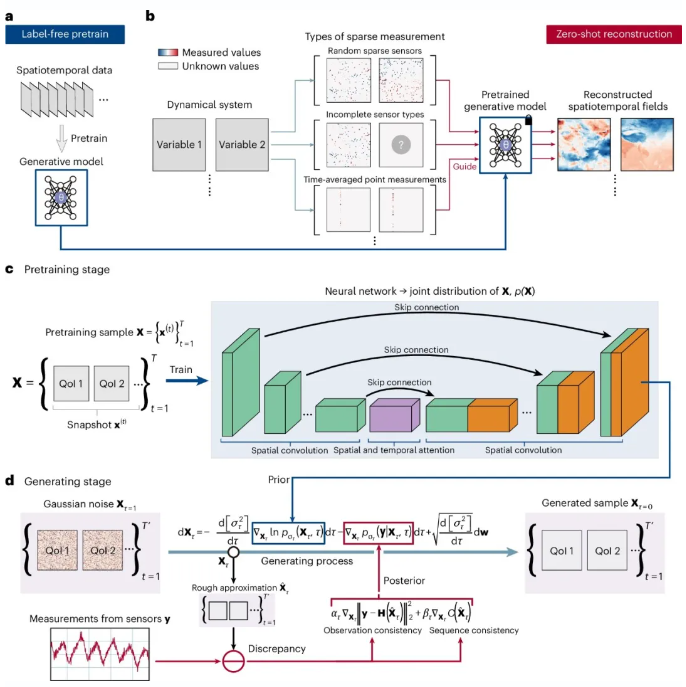
Schematic illustration of the proposed S3GM framework
This work is supported by the National Natural Science Foundation of China, the National Key Research and Development Program of China, and the Beijing Natural Science Foundation.
Original article link: https://www.nature.com/articles/s42256-024-00938-z
Editor: Lyu Xingyun
Source: School of Astronautics