On July 16, 2018, IEEE Transactions on Pattern Analysis and Machine Intelligence, a top journal on AI and machine learning, published the latest research named “Predicting Head Movement in Panoramic Video: A Deep Reinforcement Learning Approach” by Prof. Wang Zulin and Associate Professor Xu Mai from the School of Electronic and Information Engineering. Other authors include Song Yuhang, Wang Jianyi and Qiao Minglang, who are undergraduates enrolled in 2014. Beihang University is the only institute that contributes to the research.
The Inspiration in Making Standards
The team led by Associate Professor Xu has been working on standardizing virtual reality (VR) for a long time. Up to now, the team has participated in four conferences on this topic with multiple proposals being adopted as the international standards. The most significant part in VR is the processing and transmission of panoramic videos. Panoramic videos display a view in every direction. It is realized by recording the view from different perspectives and stitching the images. Panoramic videos require considerably high resolution (8K or even 16K) to achieve a fully immersive experience.
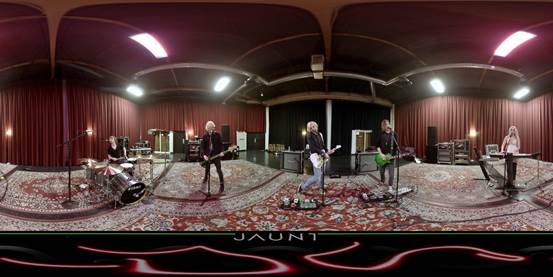
Fig. 1 A panoramic video
Associate Professor Xu thought that stereographic projection and user experience were the two main branches of VR standards. Therefore, his team was dedicated to improving user experience and solving relevant practical problems. The processing of videos with high resolution consumes a large amount of computing resources and requires much communication bandwidth, so a key issue in the researches of panoramic video and VR is to reduce the scale of data processed and transferred and improve user experience while maintaining the video quality. Xu Mai therefore led his team to study the issue.
Use Strengths to Address Issues
There are billions of cone cells and rod cells in human’s eyes, which are equivalent to a billion-pixel camera, but there are only tens of thousands of ganglion cells connecting the eyes to the brain. Thus, the data transfer rate is only 8Mbps, suggesting that not all the image data are processed by the brain. Those zones being processed are called the perception zones. Therefore, modeling human attention on panoramic video based on the working mechanism of human’s eyes becomes a significant part of the research.
Panoramic videos provide immersive and interactive experience by enabling humans to control the field of view through head movement. Thus, predicting head movement plays a key role in modeling human attention on panoramic video. Their paper establishes a database collecting the head movement and eyes movement of 58 subjects in 76 panoramic video sequences. After four months of data cleaning and processing, the heat map of each panoramic frame is generated by tracking the head movement data. The research is the first to reveal the visual mechanism of human when they are watching panoramic videos.
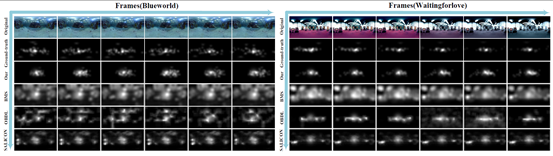
Fig. 2 Head movement maps of several frames selected from two test sequences in our panoramic video sequences-head movement database
After analyzing the heat map, the team found that although different people had different perception zones when they watched the same video content, the two things were closely interrelated. Based on this, people’s head movement can be predicted by building a prediction model whose mechanism is essentially correlative to the reinforcement learning approach. Therefore, they proposed a new deep reinforcement learning (DPL) approach to predict human’s behaviors such as head movement positions when watching panoramic video. The approach is effective in both offline and online prediction. The experiment finally validated that the heap map predicted by the algorithm proposed in the paper was much more accurate than that predicted by the traditional one, with the CC (correlation coefficient) value increasing more than 20%. The attention model can be extensively utilized in many areas of panoramic video, such as region-of-interest compression and rendering. It also offers theoretical support in improving the user experience of panoramic videos.
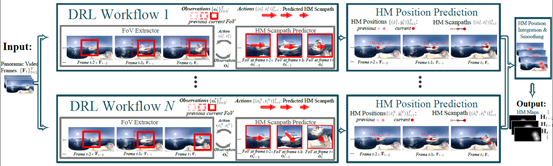
Fig. 3 Overall framework of the offline deep reinforcement learning based head movement prediction approach
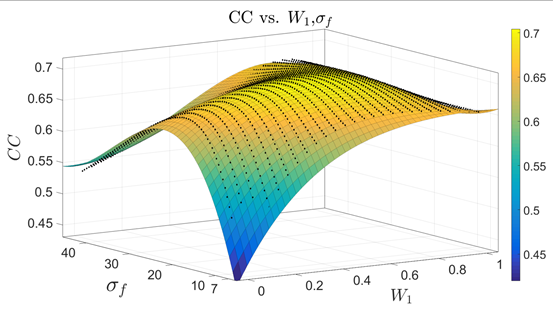
Fig.4 The fitting surface of CC results between the predicted and ground-truth head movement maps at varying combination weights and standard deviation
The Research Conducted by Undergraduates with Unremitting Efforts
The research was entirely conducted by three undergraduates under the guidance of Associate Professor Xu. “Undergraduates are highly motivated in scientific research and perform well in cooperation. Although their work seems to be less convincing than other contributors considering their academic degrees, the results turn out to be good.” Xu Mai spoke highly of the team. The three students said that Associate Professor Xu responsibly helped them solve every single problem they encountered in the research. They often received rapid and detailed replies even when the questions were asked at one or two o’clock in midnight. They held meeting each week to discuss the research progress, problems they encountered and the next steps. Associate Professor Xu encouraged them to make continuing efforts when they were told to revise the paper drastically. He helped the team modify the paper for several times before the paper was finally accepted.
Conclusions
New ideas and perspectives will lead to new reforms. Associate Professor Xu and his team successfully modeled the human attention in panoramic video taking advantage of various new approaches including deep learning and reinforcement learning. They achieved the compression of computing resources and communication bandwidth, which offered favorable conditions for the large-scale practical application of VR. The team will go deeper in the research, gradually solve the problems in practical use and improve user experience. Besides panoramic videos, Xu Mai and his team are also conducting research in improving user experience in other areas such as images and common videos. It is believed that they will make further breakthroughs in this field in the future.
The paper was funded by Natural Science Foundation of China and the Youth Talent Support Program of Beihang University.
The paper published in IEEE: https://ieeexplore.ieee.org/document/8418756/
More information about the project: https://github.com/YuhangSong/DHP
Written by Zeng Jiaqi and Cao Jiahui
Interviewed by Zeng Jiaqi
Designed by Yang Yanzhuo
Translated by Xiong Ting
Reviewed and Released by GEOOS
Please send contributions to geoos@buaa.edu.cn